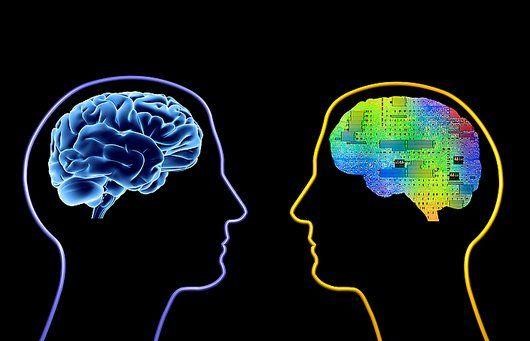
Revised 11 April 2019
Artificial intelligence (AI)--the ability of machines to learn--traces its modern roots back more than half a century. The subsequent passage of less than a decade saw a derivative field emerg: the ability of machines to teach.
Over the years, the fits and starts of machine teaching paralleled those of machine learning. Fast forward to today, however, and both stand on the cusp of enabling profound change.
The MIT Integrated Learning Initiative's mission concerns itself with improving the effectiveness of human learning. This concept paper explores the landscape of how AI might aid in that endeavor.
Consider a hypothetical "LEARNERai" that understands a person for the purpose of delivering relevant learning at the time of need. Assume that privacy is addressed--the LEARNERai serves solely on behalf of an aware learner and not, for instance, at the direction of the learner's family or school or place of employment in ways that might affect the learner's current and future employment. The LEARNERai will not, however, be limited to knowing only about its own learner; it will also draw--anonymously and securely--on the LEARNERai-enhanced learning experiences of others. For illustrative purposes, limit the scenario to that of an employee in the workplace. What might this LEARNERai need to know?
-
Basic physiological information via biosensors
-
Heart rate, eye gaze, pupil dilation, galvanic skin response, skin temperature, attention, ...
-
-
Interests via direct survey
-
Technical topics, operational topics, financial topics, leadership topics, plus personal topics ...
-
-
What you're working on right now
-
Subject matter, mode of receiving/expressing, individual/group, ...
-
-
Current work role via job description and email, text, phone, and web monitoring
-
Roles, projects, tasks, calendar, ...
-
-
Career, volunteer, and other service history via resumes, LinkedIn, and other records
-
Roles, projects, tasks, ...
-
-
Learning history via direct survey, calendars, stored credentials, and more
-
Degrees, programs, courses, conferences, books, articles, webinars, ...
-
-
Your desired career future via direct survey
-
Fields, roles, ...
-
-
Desired learning future via direct survey
-
Domains, knowledge, skills, ...
-
With that information (and more, no doubt) in place, the LEARNERai will be in a position to help optimize learning across MITili's learner/instruction/policy framework as outlined below. The concept paper will then touch on how the development of a learnerAI might intersect with MITili's learning effectiveness research mission before ending with a summary.
Learner
If a learner has the right prior knowledge, motivation, interest, and physiological readiness, he or she will be in a good position to learn. The learner isn’t always in the best position to judge the state of these conditions, however.
The LEARNERai can help.
-
Prior knowledge: the LEARNERai will use information about the learner’s prior expertise and learning experiences, coupled with the learner's confidence in that expertise and experience, to make appropriate matches with content based on the LEARNERai’s assessment of the level of that content.
-
Motivation: as used here, motivation reflects external reasons for learning—to earn a credential, receive a promotion, or otherwise further a career. The LEARNERai will provide motivating context to the learner by mapping the content of a learning experience to current and future roles of importance to the learner.
-
Interest: in comparison with motivation, interest is intrinsic--typically, a learner either does or does not like a topic, although interest may certainly increase with familiarity and expertise. The LEARNERai will draw on learner-expressed interests to match with the content in a learning experience when skill or process (and not content) is the primary learning objective.
-
Physiological readiness: The learner needs to be well-rested, well-fed, otherwise in good physical shape, and in a good mental state. The LEARNERai will detect these conditions and align learning experiences with the learner’s physiological receptiveness.
Instruction
If the instruction has the right content, delivery, and assessment, the odds of effective learning improve.
The LEARNERai can help.
-
Content: Content includes the breadth, depth, and accuracy of the subject matter and the production value with which the subject matter is configured. Content may cover knowledge or skills. The LEARNERai, knowing a lot about what’s relevant to the learner, will uncover content addressing one of three needs: when the learner knows that content is needed; when the learner’s manager, peers, or subordinates know that content is needed; and most important, when no one is aware that content is needed.
-
Delivery: Delivery variables include human vs. digital, synchronous vs. asynchronous, duration of learning, user-requested vs. pushed-to-user, and device through which the learning is consumed. The LEARNERai will look at past successes and current needs to help deliver content when it’s most valuable. That timing might be in advance of a learning need (“learning before performing”) or in just-in-time fashion at the time of need (“learning while performing”).
-
Assessment: Assessment has to do with the frequency and depth with which the learner is assessed and the formative manner in which the responses are used to guide the next piece of content and delivery. A robust LEARNERai will add fidelity and precision to the adaptive-branching inherent in effective “What’s next?” learning. Moreover, the learnerAI will track, record, analyze, and provide feedback on learning, both formal and informal, based on the learner’s creations, responses, actions, calendar, and other inputs.
Policy
If the policy has the right law, access, funding, leadership, and measurement, the prospects for effective learning are better still.
The LEARNERai can help.
-
Law, access, and funding: Laws and regulations must be conducive to learning. Learners must have access to learning. This isn’t access via funding, but rather, access via circumstance. For instance, a job role that isn’t eligible for a particular type of training or isn’t in the right location for that training isn’t helped regardless of how good the training is. Finally, funds have to be available to pay for the learning experience. These funds may be provided by the learner or the provider of instruction. These three elements of the framework are included for completeness, but don’t not lend themselves strongly to improvement via AI.
-
Leadership: Leaders of those providing instruction must have a philosophy conducive to/supportive of learning. Here, the LEARNERai can be tuned, and tune itself, to learning opportunities (content, delivery, and assessment) in line with executive mission statements and vision and management implementations of those missions and visions. Moreover, leadership could draw on anonymized and aggregated feedback from all of the LEARNERai instances within the organization to refine its learning vision and gaps therein.
-
Measurement: Measurement as used here is different from the formative level of assessment guiding the learner’s next instruction. Rather, it addresses the summative level of what learners come to know, how their behaviors change, and what organizational improvements those behavior changes drive. The LEARNERai, through its tracking and reporting, will assist learners in demonstrating the value to their employer of the learning they undergo, thereby establishing a case to be made for future learning.
The research angle
MITili’s mission suggests a path forward that includes not just the development of AI designed to accomplish some or all of the LEARNERai capabilities outlined above, but also performing the research to demonstrate that the presence of the LEARNERai yields better learning outcomes than more traditionally supported learning.
SUMMARY
Learners will equip themselves with a LEARNERai guide. Ideally, the learner will own and control the LEARNERai the way they own a LinkedIn account, for instance. The LEARNERai will not be provided by--and therefore be accessible by--the learner’s employer. While learners may still feel pressure to share LEARNERai data with employers and especially prospective employers, the learner ownership will mitigate the intrusiveness.
The learner will own, control, and tune the LEARNERai to monitor physiology, job descriptions, to-do lists, communications, actions, and more. As a result, the LEARNERai will assist with desired, assigned, and unanticipated learning. This learning will be delivered optimally: when the learner is ready to learn and/or when the learning is of the most value. In the background, the LEARNERai will record learning for the learner to share when that sharing best benefits the learner.