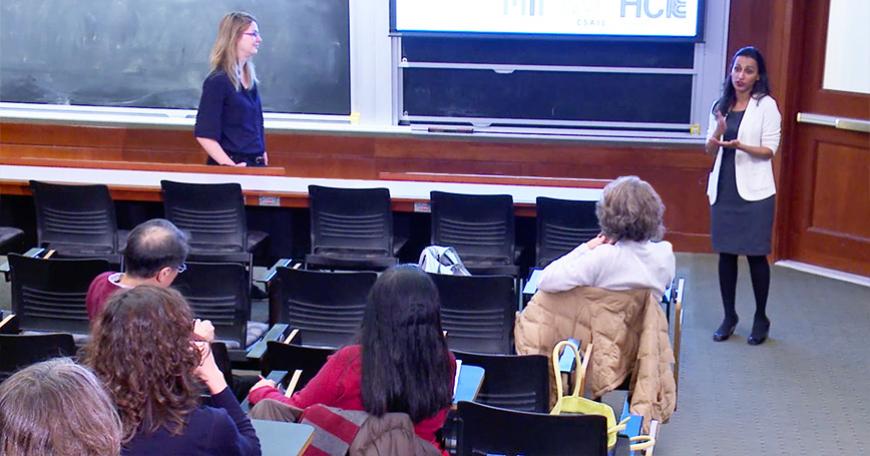
While it seems one never forgets how to ride a bike, the learning process can be long and frustrating: training wheels, wobbly handlebars, and skinned knees. For many, making the transition from walking on solid ground to cruising on two wheels can be quite daunting. Learning any skill, for that matter, seems to entail a period of discomfort and struggle before it becomes second nature.
Because of this, Professor Stefanie Mueller and PhD student Dishita Turakhia have been exploring ways to improve the learning process. In their xTalk on November 7, they demonstrated their advances at replicating adaptive learning, an effective technique designed to personalize learning to each student, in the physical realm. As their central idea, they strive to maintain task difficulty at the optimal challenge point: not too hard, not too easy. While this idea has been used in learning concepts and languages, it has been challenging to implement for physical skills, which Mueller and Turakhia seek to do.
To elucidate the concepts, they introduced two new terms: nominal and functional task difficulty. Nominal difficulty is objective: playing a game in easy vs. hard mode, or shooting a 3-pointer vs. making a lay-up. Functional difficulty, however, is dependent on the participant. To a skilled player, “hard” may pose no challenge, but to a beginner, “easy” may be all but impossible.
With these ideas in mind, Turakhia performed a series of user studies using shooting baskets as a medium. In the studies, an automated system could vary both height of the basket and radius of the hoop in response to the participant’s performance. Having split the participants into two groups, Turakhia then compared the relative improvement of those who used the automated system and those who merely practiced on a static hoop. The adaptive group improved significantly more than the static group, verifying the effectiveness of maintaining the optimal challenge point.
Interestingly, Turakhia then allowed the participants in the adaptive group to choose the height and radius themselves, whereas these parameters had been previously automatically determined. Again, comparing relative improvement with those who used a static hoop, the adaptive group still performed better; however, the improvements fell short of those who used the automated system. According to Turakhia, this is evidence for a key human shortcoming: that humans are generally inept at self-assessment.
Transitioning to more general training tools, Turakhia described a program that allows developers to create their own systems, with a myriad of options for sensors, adjustments, and learning curves. With this interface, for example, one could replicate the training program used in Turakhia’s basketball study.
Finally, Prof Mueller introduced an exciting future direction: creating games that build maker skills by incorporating real-life construction in the gameplay. As a concrete example, when a certain item is needed in the game, instructions are given to the player to construct the prop in real life. When it is properly executed, the player can return to the virtual world and begin using the prop, having gained valuable maker experience. In collaboration with the MIT Game Lab and the NuVu Innovation School, Mueller will continue to explore this exciting new path.