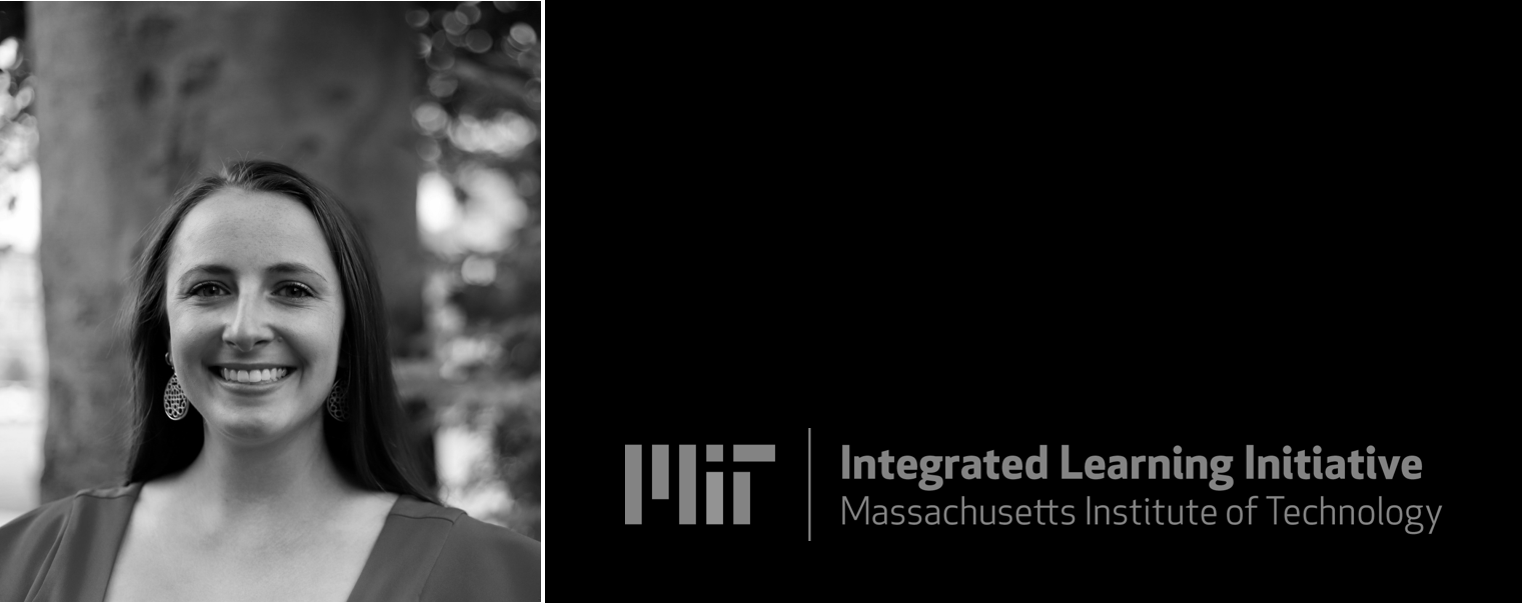
Lauren is a Learning Engineer on the Residential Education team at MIT Open Learning. Her work on the team involves helping faculty and course teams design, develop, assess, and iteratively improve digital learning experiences, with a focus on incorporating research-based teaching practices into the design of courses that leverage MIT-wide educational technologies such as Canvas.
Lauren has a MA in Learning Engineering from Boston College’s Lynch School of Education & Human Development and was a student in the inaugural cohort of the program. Combining this with a former career in technical training and consulting, she sees herself as working at the intersection of technology and pedagogy.
What exactly is a learning engineer?
A learning engineer applies the learning sciences and uses human-centered engineering design methodologies and data-informed decision making to support learners and their development. In my MIT-specific context on the Residential Education team, this means working with instructors to design learning experiences, typically involving technology, and helping them iterate upon that design using data and research-based insights.
While I have the title “learning engineer” as an individual, as a practice and a process, learning engineering also involves different skills and areas of expertise being distributed across members of a team who are all involved in the design of a learning experience – for example, those with technical expertise in a specific tool, data science, subject matter, etc. So the term “learning engineering” can apply to a team driving this work, not just an individual.
What do you think is the most important aspect of the future of education?
Continuous iteration and improvement! This is a hallmark of learning engineering. The pandemic context of the last couple of years forced many instructors to reevaluate their teaching and change their strategies and practices. And some of us can’t help but wonder: would they still have made these changes or focused more on their teaching practices had it not been for the pandemic situation forcing remote and online learning? I envision a future where instructors are encouraged and supported to incrementally iterate and experiment with new innovations in their teaching, being prompted to do so by things like student feedback, data, and professional development opportunities where they learn and discuss new ideas in teaching and learning.
How does data analysis drive MIT’s education effectiveness?
Data analysis enables improvement and optimization of a learning environment. Data can explain certain phenomena we might witness through other observations about a learning experience, and helps us follow up with and address what we are seeing. The data we collect can also tell a story, and once we discover that story through analysis, we can operationalize the findings and patterns to make a learning experience even more effective. Similar to how applying principles and methods from the learning sciences helps us design for learners and learning, data helps us understand those learners’ experiences in a certain context, such as how they interact with MOOCs and other digital technologies, or how they collaborate with peers.
What is your favorite thing about working at MIT?
My immediate thought was: getting to work with MIT faculty and instructors. Narrowly, this includes things I get to do in my role like consulting with faculty on edtech tools and sharing ideas about teaching and learning. But upon further reflection, it’s much broader than that. I think it’s getting to experience everyone in the MIT community consulting with each other and sharing ideas with each other, combining faculty, staff, and student voices. I am so energized by all of the initiatives there are to amplify the great work and innovation being tackled at MIT and the opportunities to share lessons learned and key insights.