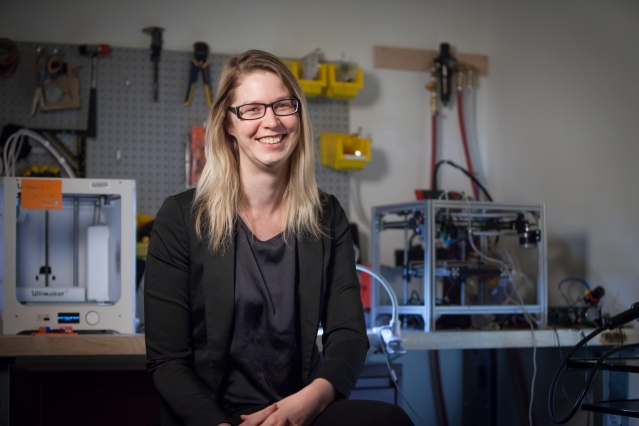
Stefanie Mueller is an assistant professor in the MIT Electrical Engineering and Computer Science (EECS) department and a member of the Computer Science and Artificial Intelligence Laboratory (CSAIL). In her research, she develops novel hardware and software systems that advance personal fabrication technologies. Stefanie publishes her work at the most selective HCI venues CHI and UIST and has received a best paper award and two best paper nominees in the past. She is also serving on the CHI and UIST program committees as an associate chair. In addition, Stefanie has been an invited speaker at universities and research labs, such as Harvard, Stanford, UC Berkeley, CMU, Microsoft Research, Disney Research, and Adobe Research.
When did you first become interested in adaptive learning?
My research group works in the area of personal fabrication, i.e. we investigate how to leverage 3D printing and related technologies to personalize products for each user’s individual needs. Since learning needs a lot of customization based on each learner’s strengths, weaknesses, and pace, it was a natural extension of our work on personalization.
What is your vision for adaptive learning systems?
While investigating personalized learning systems closer, we noticed that there is a lot of work on digital adaptive learning, i.e. there are many platforms that allow learners to acquire skills in, e.g., foreign languages and math by presenting them with learning tasks that are adapted to their current skill level.
However, to date, adaptive learning systems have not been explored in the context of learning motor skills that involve physical tools, such as those that need to be mastered during childhood development (riding a bike, writing with a pen), in the workplace (e.g. specific drills or assembly tools in factories), during rehabilitation (e.g., handling crutches), and for learning sports and the arts (e.g., rock climbing, learning how to play the violin).
How exactly will those adaptive physical tools work?
Using sensors integrated with the tool, we will measure how well the learner is progressing. For instance, while a child learns how to ride a bike, we can measure the child’s skill level using sensors on the training wheels that detect how much the child is relying on them to balance. Actuators then change the difficulty level, i.e. in the case of the bike, motors can lift or lower the training wheels to make it harder or easier to balance.
What advice do you have for students in junior high or high school interested in engineering and computer science?
Once you get into college, try many different classes and undergraduate research experiences early on in your studies to find an area you are passionate about—then spend the last years of your degree program to go in-depth in your area of choice. Working 6-12 months closely with a research group and contributing a substantial piece of work is worth a lot more than doing many 1-2 month internships.
What is your favorite thing about working at MIT?
The best part of MIT are the people—everyone is excited about being here and contributing to this incredible place, you can literally feel the energy in the room.